In this blog post, I will show you how we can use basic graph analysis metrics to analyze Big Structures. I will do the analysis of the UMBEL reference concept structure to demonstration how graph and network analysis can be used to better understand the nature and organization of possible issues with Big Structures such as UMBEL. | ![]() |
I will first present the formulas that have been used to perform the UMBEL analysis. To better understand this section, you will need some math knowledge, but there is nothing daunting here. You can probably safely skip that section if you desire.
The second section is the actual analysis of a Big Structure (UMBEL) using the graph measures I presented in the initial section. The results will be presented, analyzed and explained.
After reading this blog post, you should better understand how graph and network analysis techniques can be used to understand, use and leverage Big Structures to help integrate and interoperate disparate data sources.
[extoc]
Big Structure: Big in Size
A Big Structure is a network (a graph) of inter-related concepts which is composed of thousands or even hundred of thousands of such concepts. One characteristic of a Big Structure is its size. By nature, a Big Structure is too big to manipulate by hand and it requires tools and techniques to understand and assess the nature and the quality of the structure. It is for this reason that we have to leverage graph and network measures to help us in manipulating these Big Structures.
In the case of UMBEL, the Big Structure is a scaffolding of reference concepts used to link external (unrelated) structures to help data integration and to help unrelated systems inter-operate. In a World where the Internet Of Things is the focus of big companies and where there are more than 400 standards, such techniques and technologies are increasingly important, otherwise it will end-up being the Internet of [Individual] Things. Such a Big Structure can also be used for other tasks such as helping machine learning techniques to categorize and disambiguate pieces of data by leveraging such a structure of types.
UMBEL as a Graph
UMBEL is an RDF and OWL ontology of a bit more than 26 000 reference concepts. Because the structure is represented using RDF, it means that it is a directed graph. All of UMBEL’s vertices
are classes, and all of the edges
are properties.
The most important fact to keep in mind until the end of this blog post is that we are manipulating a directed graph. This means that all of the formulas used to analyze the UMBEL graph are formulas applicable to directed graphs only.
I will keep the normal graph analysis language that we use in the literature, however keep in mind that a vertice
is a class
or a named individual
and that a edge
is a property
.
The UMBEL structure we are using is composed of the classes view
and the individuals view
of the ontology. That means that all the concepts are there, where some of them only have a class view, others have an individual view and others have both (because they got punned).
Graph Analysis Metrics
In this section, I will present the graph measures that we will use to perform the initial UMBEL graph analysis. In the next section, we will make the analysis of UMBEL using these measures, and we will discuss the results.
This section uses math notations. It could be skipped, but I suggest to try to take time some time to understand each measure since it will help to understand the analysis.
Some Notations
In this blog post, a graph is represented as where
is the graph,
is the set of all the vertices and
is the set of all the edges of the same type that relates vertices.
The UMBEL analysis focuses on one of the following transitive properties:Â rdfs:subClassOf
, umbel:superClassOf
, skos:broaderTransitive
, skos:narrowerTransitive
and rdf:type
. When we do perform the analysis, we are picking-up a subgraph that is composed of all the connections between the vertices that are linked by this edge (property).
Density
The first basic measure is the density
of a graph. The density measures how many edges are in set compared to the maximum possible number of edges between vertices in set
. The density is measured with:
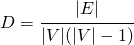
where is the
density
, is the number of
properties (edges)
and is the number of
classes (vertices).
The density is a ratio of the number of edges that exists, and the number of edges that could exists in the graph. is the number of edges in the graph and
is the number of possible maximum number of edges.
The maximum density is 1, and the minimum density is 0. The density of a graph gives us an idea about the number of connections that exists between the vertices.
Average Degree
The degree
of a vertex is the number of edges that connect that vertex to other vertices. The average degree of a graph is another measure of how many edges are in set
compared to number of vertices in set
.

where is the
average degree
, is the number of
properties (edges)
and is the number of
classes (vertices).
This measure tells the average number of nodes to which any given node is connected.
Diameter
The diameter
of a graph is the longest shortest path between two vertices in the graph. This means that this is the longest path that excludes all detours, loops, etc. between two vertices.
Let be the length of the shortest path between
and
. And
. The diameter of the graph is defined as:

This metric gives us an assessment of the size of the graph. It is useful to understand the kind of graph we are playing with. We will also relate it with the average path length to assess the span of the graph and the distribution of path lengths.
Average Path Length
The average path length is the average of the shortest path length, averaged over all pairs of vertices. Let be the length of the shortest path between
and
. And
.

where, is the number of vertices in the graph
; where,
is the number of pairs of distinct vertices. Note that the number of pairs of distinct vertices is equal to the number of shortest paths between all pairs of vertices if we pick just one in case of a tie (two shortest paths with the same length).
In the context of ontology analysis, I would compare this metric as the speed of the ontology. What I mean by that is that one of the main tasks we do with an ontology is to infer new facts from known facts. Many inferring activities requires traversing the graph of an ontology. This means that the smaller the average path length between two classes, the more performant these inferencing activities should be.
Average Local Clustering Coefficient
The local clustering coefficient quantifies how well connected the neighborhood vertices of a given vertex are. It is the ratio of the edges that exists between all of the neighborhood vertices of a given vertex and the maximum number of possible edges between these same neighborhood vertices.

where is the number of neighborhood vertices,
is the maximum number of edges between the neighborhood vertices and
is the set of all the neighborhood vertices for a given vertex
.
The local clustering coefficient is represented by the sum of the clustering coefficient of all the vertices of a graph
divided by the number of vertices in
. It is given by:

Betweenness Centrality
Betweenness centrality is a measure of importance of a node in a graph. It is represented by the number of times a node participates in the shortest path between other nodes. If a node participates in the shortest path of multiple other nodes, then it means that it is more important than other nodes in the graph. It acts like a conduit.
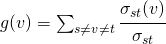
where is the total number of shortest paths from node
to node
and
is the number of those paths that pass through
.
In the context of ontology analysis, the betweenness centrality will tell us which of the classes that participates the more often in the shortest paths of a given transitive property between other classes. This measure is interesting to help us understand how a subgraph is constructed. For example, if we take a transitive property such as rdfs:subClassOf,
then the graphs generated by a subgraph composed of this relationship only should be more hierarchic by the semantic nature of the property. This means that the nodes (classes) with the highest betweenness centrality value should be classes that participate in the upper portion of the ontology (the more general classes). However, if we think about the foaf:knows
transitive property between named individuals, then the results should be quite different and suggest a different kind of graph.
Initial UMBEL Graph Analysis
Now that we have a good understanding of some core graph analysis measures, we will use them to analyze the graph of relationship between the UMBEL classes and reference concepts using the subgraphs generated by the properties: rdfs:subClassOf
, umbel:superClassOf
, skos:broaderTransitive
, skos:narrowerTransitive
and rdf:type
.
Density
The maximum number of edges in UMBEL is: which is about two thirds of a billion of edges. This is quite a lot of edges, but it is important to keep in mind since most of the following ratios are based on this maximum number of edges (connections) between the nodes of the UMBEL graph.
Here is the table that shows the density of each subgraph generated by each property:
Class view | Individual view | ||||
Metric | sub class of | super class of | broader | narrower | type |
Number of edges | 39 410 | 116 792 | 36 016 | 36 322 | 271 810 |
Density | 0.0000567 | 0.0001402 | 0.0000518 | 0.0000523 | 0.0001021 |
As you can see, the density of any of the UMBEL subgraphs is really low considering that the maximum density of the graph is 1. However this gives us a picture of the structure: Most of the concepts have no more than few connections between each node for any of the analyzed properties.
This makes sense, since a conceptual structure is meant to model relationships between concepts that represent concepts of the real world, and in the real world, the concepts that we created are far from being connected to every other concept.
This is actually what we want: we want a conceptual structure which has a really low density. This suggest that the concepts are unambiguously related and hierarchized.
Having a high density (let’s say, 0.01
, which would mean that there are nearly 7 million connections between the 26 345 concepts for a given property) may suggest that the concepts are too highly connected which could suggest that using the UMBEL ontology for tagging, classifying and reasoning over its concepts won’t be an optimal choice because of the nature of the structure and its connectivity (and possible lack of hierarchy).
Average Degree
The average degree shows the average number of UMBEL nodes that are connected to any other node for one of the given property.
Class view | Individual view | ||||
Metric | sub class of | super class of | broader | narrower | type |
Number of Edges | 39406 | 97323 | 36016 | 36322 | 70921 |
Number of Vertices | 26345 | 26345 | 26345 | 26345 | 26345 |
Average degree | 1.4957 | 3.6941 | 1.3670 | 1.3787 | 2.6920 |
As you can see, the number are quite low: from 1.36
to 3.69
. This is consistent with what we saw with the density
measure above. This helps confirm our assumption that all of these properties create mostly hierarchical subgraphs.
However there seems to be one anomaly with these results, the average degree 3.69
of the umbel:superClassOf
property. Intuitively, its degree should be near the one of the rdfs:subClassOf
but it is far from this: it is more than twice its average degree. Looking at the OWL serialization of the UMBEL version 1.05 reveals that most umbel:RefConcept
do have 3 triples:
umbel:superClassOf skos:Collection ,
skos:ConceptScheme ,
skos:OrderedCollection .
This makes no sense that the umbel:RefConcept
are super classes of these skos
classes. I suspect that this got introduced via punning
at some point in the history of UMBEL and got unnoticed until today. This issue will be fixed in a coming maintenance version of UMBEL.
If we check back the density measure
of the graph, we notice that we have a density of 0.0001402
for the umbel:superClassOf
property versus 0.0000567
for the rdfs:subClassOf
property which has about the same ratio. So we could have noticed the same anomaly by taking a better look at the density measure
.
But in any case, this shows how this kind of graph analysis can be used to find such issues in Big Structures (structures too big to find all these issues by scrolling the code only).
Diameter
The diameter of the UMBEL graph is like the worse case scenario
. It tells us what is the longest shortest path for a given property’s subgraph.
Class view | Individual view | ||||
Metric | sub class of | super class of | broader | narrower | type |
Diameter | 19 | 19 | 19 | 19 | 4 |
In this case, this tell us that the longest shortest path between two given nodes for the rdfs:subClassOf
property is 19. So in the worse case, if we infer something between these two nodes, then our algorithms will require maximum 19 steps (think in terms of a breath first search, etc).
Average Path Length Distribution
The average path length distribution shows us the a number paths that have x
in length. Because of the nature of UMBEL and its relationships, I think we should expect a normal distribution. An interesting observation we can do is the the average path length is situated around 6, which is the six degree of separation.
We saw that the “worse case scenario” was a shortest path of 19 for all the property except rdf:type
. Now we know that the average is around 6.
rdfs:SubClassOf
umbel:superClassOf
Here we can notice an anomaly in the expected normal distribution of the path lengths. Considering the other analysis we did, we can consider that the anomaly is related to the umbel:superClassOf
issue we found. What we will have to re-check this metric once we fix the issue. I expect we will see a return to a normal distribution.
skos:broaderTransitive
skos:narrowerTransitive
rdf:type
Average Local Clustering Coefficient
The average local clustering coefficient will tell us how clustered the UMBEL subgraphs are.
Class view | Individual view | ||||
Metric | sub class of | super class of | broader | narrower | type |
Average local clustering coefficient | 0.0001201 | 0.00000388 | 0.03004554 | 0.00094251 | 0.77191429 |
As we can notice, UMBEL does not have the small-world effect with its small clustering coefficients depending on the properties we are looking at. It means that there is not a big number of hubs in the network and so that the number of steps from going from a class or a reference concept to another is higher than in other kinds of networks, like airport networks. This makes sense by looking at the average path length and the path length distributions we observed above.
At the same time, this is the nature of the UMBEL graph: it is meant to be a a well structured set of concepts with multiple different specificity layers.
To understand its nature, we could consider the robustness of the network. Normally, networks with high average clustering coefficients are known to be robust, which means that if a random node is removed, it shouldn’t impact the average clustering coefficient or the average path length of that network. However, in a network that doesn’t have the small-world effect, then they are considered less robust which means that if a node is removed, it could greatly impact the clustering coefficients (which would be lower) and the average path length (which would be higher).
This makes sense in such a conceptual network: if we remove a concept from the structure, it will most than likely impact the connectivity of the other concepts of the network.
One interesting thing to notice is the clustering coefficient of 0.03
for the skos:broaderTransitive
property and 0.00012
for the rdfs:subClassOf
property. I have no explanation for this discrepancy at the moment, but this should be investigated after the fix as well since intuitively these two coefficient should be close.
Betweenness Centrality
As I said above, In the context of ontology analysis, the betweenness centrality tells us which of the classes participates more often in the shortest paths of a given transitive property between other classes. This measure is useful to help us understand how a subgraph is constructed.
If we check the results below, we can see that all the top nodes are nodes that we could easily classify as being part of the upper portion of the UMBEL ontology (the general concepts). Another interesting thing to notice is that the issue we found with the umbel:superClassOf
property doesn’t seem to have any impact on the betweenness centrality of this subgraph.
rdfs:subClassOf
PartiallyTangible | 0.1853398395065167 |
HomoSapiens | 0.1184076250864128 |
EnduringThing_Localized | 0.1081317879905902 |
SpatialThing_Localized | 0.092787668995485 |
HomoGenus | 0.07956810084399618 |
You can download the full list from here
umbel:superClassOf
PartiallyTangible | 0.1538140444614064 |
HomoSapiens | 0.1053345447606491 |
EnduringThing_Localized | 0.08982813055659158 |
SuperType | 0.08545549956197795 |
Animal | 0.06988077993945754 |
You can download the full list from here
skos:broaderTransitive
PartiallyTangible | 0.2051286996513612 |
EnduringThing_Localized | 0.1298479341295156 |
HomoSapiens | 0.09859060900526667 |
Person | 0.09607892589570508 |
HomoGenus | 0.08806468362881092 |
You can download the full list from here
skos:narrowerTransitive
PartiallyTangible | 0.2064665713447941 |
EnduringThing_Localized | 0.1294085106511612 |
HomoSapiens | 0.1019893654646639 |
Person | 0.09366129700219764 |
HomoGenus | 0.09047063411213117 |
You can download the full list from here
rdf:type
owl:Class | 0.3452564052004049 |
RefConcept | 0.3424169525837704 |
ExistingObjectType | 0.0856962574437039 |
ObjectType | 0.01990245954437302 |
TemporalStuffType | 0.01716817183946576 |
You can download the full list from here
Analysis with Inferred UMBEL
Now, let’s push the analysis further. Remember that I mentioned that all the properties we are analyzing in this blog post are transitive? Let’s perform exactly the same metrics analysis, but this time we will use the transitive closure
of the subgraphs.
The transitivity characteristic of a property is simple to understand. Let’s consider this tiny graph where the property
is a transitive property. Since
is transitive, there is also a relationship
.
Given we inferred
using the transitive relation
.
Now, let’s use the power of these transitive properties and let’s analyze the transitive closure
of the subgraphs that we are using to compute the metrics. The transitive closure
is simple to understand. From the input subgraph, we are generating a new graph where all these transitive relations are explicit.
Let’s illustrate that using this small graph: . The
transitive clojure
would create a new graph:
This is exactly what we will be doing with the sub-graphs created by the properties we are analyzing in this blog post. The end result is that we will be analyzing a graph with many more edges than we previously had with the non transitive closure versions of the subgraphs.
What we will analyze now is the impact of considering the transitive closure upon the ontology metrics analysis.
Density
Remember that the maximum number of edges in UMBEL is: , which is about two thirds of a billion edges.
Class view | Individual view | ||||
Metric | sub class of | super class of | broader | narrower | type |
Number of Edges | 789 814 | 922 328 | 674 061 | 661 629 | 76 074 |
Density | 0.0011380 | 0.0013289 | 0.0009712 | 0.0009533 | 0.0001096 |
As we can see, we have many more edges now with the transitive closure. The density of the graph is higher as well since we inferred new relationships between nodes from the transitive nature of the properties. However it is still low considering the number of possible edges between all nodes of the UMBEL graph.
Average Degree
We now see the impact of transitive closure on the average degree of the subgraphs. Now each node of the subgraphs are connected by 25 to 35 other nodes in average.
Class view | Individual view | ||||
Metric | sub class of | super class of | broader | narrower | type |
Number of Edges | 789 814 | 922 328 | 674 061 | 661 629 | 76 074 |
Number of Vertices | 26345 | 26345 | 26345 | 26345 | 26345 |
Average degree | 29.97965 | 35.00960 | 25.58591 | 25.11402 | 2.887606 |
One interesting fact is that the anomaly disappears with this transitive closure subgraph for the umbel:superClassOf
property. There is still a glitch with it, but I don’t think it would raise suspicion at first. This is important to note since we won’t have noticed this issue with the current version of the UMBEL ontology if we would have analyzed the transitive closure of the subgraph only.
Diameter
Class view | Individual view | ||||
Metric | sub class of | super class of | broader | narrower | type |
Diameter | 2 | 2 | 2 | 2 | 2 |
As expected, the diameter of any of the transitive closure subgraphs is 2
. It is the case since we made explicit a fact (a edge) between two nodes that was not explicit at first. This is good, but this is no quite useful from the perspective of ontology analysis.
This would only be helpful if the number were not 2
which would suggest some errors in the way you computed the diameter of the graph.
However what we can see here is that the speed of the ontology (as defined in the Average Path Length
section above) is greatly improved. Since we forward-chained the facts in the transitive closure sub-graphs, it means that knowing if a class A
is a sub-class of a class B
is much faster, since we have a single lookup to do instead of an average of 6 for the non transitive closure version of the subgraphs.
Average Path Length Distribution
All of the path length distributions will be the same as this one:
Since the diameter is 2, then we have a full lot of paths at 2, and 26 345 at 1. This is not really that helpful from the standpoint of ontology analysis.
Average Local Clustering Coefficient
Class view | Individual view | ||||
Metric | sub class of | super class of | broader | narrower | type |
Average local clustering coefficient | 0.3281777 | 0.0604906 | 0.2704963 | 0.0138592 | 0.7590267 |
Some of the properties like the rdfs:subClassOf
property shows a much stronger coefficient than with the non-transitive closure version. This is normal since all the nodes are connected to the other nodes down the paths. So, if a node in between disappears, then it won’t affect the connectivity of the subgraph since all the linkage that got inferred still remains.
This analysis also suggests that the transitive closure version of the subgraphs are much stronger (which makes sense too).
However I don’t think this metric is that important of a characteristic to check when we analyze reference ontologies since they do not need to be robust. They are not airport or telephonic networks that need to cope with disappearing nodes in the network.
Betweenness Centrality
What the betweenness centrality measure does with the transitive closure of the subgraphs is that it highlight the real top concepts of the ontology like Thing
, Class
, Individual
, etc. Like most of the other measures, it blurs the details of the structure (which is not necessarily a good thing).
rdfs:subClassOf
SuperType | 0.03317996389023238 |
AbstractLevel | 0.02810408526564482 |
Thing | 0.02772171675862925 |
Individual | 0.02747482318621853 |
TopicsCategories | 0.02638342698407473 |
You can download the full list from here
umbel:superClassOf
The interesting thing here is that this measure actual shows the actual concepts for which we discovered an issue with above.
skos:Concept | 0.02849203320293865 |
owl:Thing | 0.02849094898994718 |
SuperType | 0.02848878056396423 |
skos:ConceptScheme | 0.02825567477079737 |
skos:OrderedCollection | 0.02825567477079737 |
You can download the full list from here
skos:broaderTransitive
Thing | 0.03225428380683926 |
Individual | 0.03196350419108375 |
Location_Underspecified | 0.0261924189600178 |
Region_Underspecified | 0.02618796825161338 |
TemporalThing | 0.02594169571990208 |
You can download the full list from here
skos:narrowerTransitive
Thing | 0.03298126713602109 |
Location_Underspecified | 0.02680852092899528 |
Region_Underspecified | 0.02680398659044947 |
TemporalThing | 0.02648809433842489 |
SomethingExisting | 0.02582305801837314 |
You can download the full list from here
rdf:type
owl:Class | 0.3452564052004049 |
RefConcept | 0.342338078899975 |
ExistingObjectType | 0.0856962574437039 |
ObjectType | 0.01990245954437302 |
TemporalStuffType | 0.01716817183946576 |
You can download the full list from here
Conclusion
This blog post shows that simple graph analysis metrics applied to Big Structures can be quite helpful to understand their nature, how they have been constructed, what is their size, their impact on some algorithms that could use them, and to find potential issues in the structure.
One thing we found is that the correlation between the properties rdfs:subClassOf
and skos
:broaderTransitive
are nearly identical. They nearly have the same values for each metrics. If you were new to the UMBEL ontology you wouldn’t have known this fact without doing this kind of analysis or by spending much time looking at the serialized OWL file. It doesn’t tell us anything about how similar the relations are, but it does tell us that they have the same impact on the ontology’s graph structure.
Performing this analysis also led us to discover a few anomalies with the umbel:superClassOf
property, suggesting an issue with the current version of the ontology. This issue would have been hard to notice, and understand, without performing such a graph analysis to the structure.
However, I also had the intuition that the analysis of the transitive closure of the subgraphs would have led to more interesting results. At best that analysis did confirm a few things, but in most of the cases it only blurred the specificities of most of the metrics.
These analysis metrics will soon be made available as standard Web services, so that they may be applied against any arbitrary graph or ontology.